D-Wave Quantum Inc. (NYSE: QBTS) and the pharmaceutical division of Japan Tobacco Inc. (JT) finished a joint proof-of-concept project using quantum computing and artificial intelligence in the drug discovery process.
Announced on Thursday, JT and D-Wave augmented large language models (LLM) with a quantum-hybrid workflow and increased their generative abilities. This allowed JT to produce novel, more “drug-like” molecular structures, improving beyond those found in the training datasets for the quantum-hybrid generative AI system.
The team showed that hybrid LLM models using classical computation and D-Wave’s quantum processing unit (QPU) generated more valid molecules than classical methods alone. Molecules from QPU-assisted LLM training also scored higher for drug-likeness than both the training dataset and those from purely classical models. The QPU helped produce higher quality, lower energy samples. These results show the benefits of quantum computing in generative AI for drug discovery.
Quantum processing units explore complex chemical spaces more efficiently by sampling from a wider range of low-energy molecular states.
When integrated with large language models, QPUs guide the models toward generating chemically valid and drug-like molecules. Classical computation handles broader tasks, while QPUs focus on optimization, improving output quality and accelerating discovery in drug design workflows.
This project aims to speed up the discovery of small-molecule compounds while improving quality.
It uses D-Wave’s quantum annealing technology within JT’s AI framework to train LLMs, like the transformer behind ChatGPT, for chemical space exploration.
Read more: D-Wave Quantum declared to have quantum computing supremacy over normal computers
Read more: D-Wave Quantum sells world’s largest quantum computer to German research firm
The first instance where quantum computing outperformed LLM training
Dr. Masaru Tateno, Chief Scientific Officer of Central Pharma Research Institute, explained that their quantum-hybrid AI system produced more “drug-like” compounds than the training dataset, without needing specific molecular property inputs in the model.
He noted this is the first instance annealing quantum computation has outperformed classical methods in LLM training for drug discovery.
The results show that annealing quantum computing can provide high-quality, low-energy samples that improve generative AI models. Looking ahead, the team plans to leverage D-Wave’s quantum annealing technology to further enhance Quantum AI-driven drug discovery.
“AI has made impressive advancements but faces a computational challenge due to escalating power needs and costs,” said Dr. Alan Baratz, CEO of D-Wave.
After the proof-of-concept project, JT’s pharmaceutical division plans to advance Quantum AI-driven drug discovery technology and molecular design.
Building on the success of their proof-of-concept project, JT’s pharmaceutical division joins industry leaders like IBM (NYSE: IBM), Alphabet Inc (NASDAQ: GOOG), and Zapata Computing in advancing quantum computing for drug discovery. Their efforts aim to push the boundaries of molecular design and accelerate the development of innovative therapies.
IBM Quantum focuses on using quantum algorithms to simulate molecular interactions and accelerate drug development, especially for protein folding.
Meanwhile, Google’s Quantum AI division is exploring quantum computing’s potential in simulating complex molecular systems, aiming to enhance drug discovery.
Furthermore, Zapata Computing is developing quantum algorithms to improve molecular simulations, material science, and drug design.
.
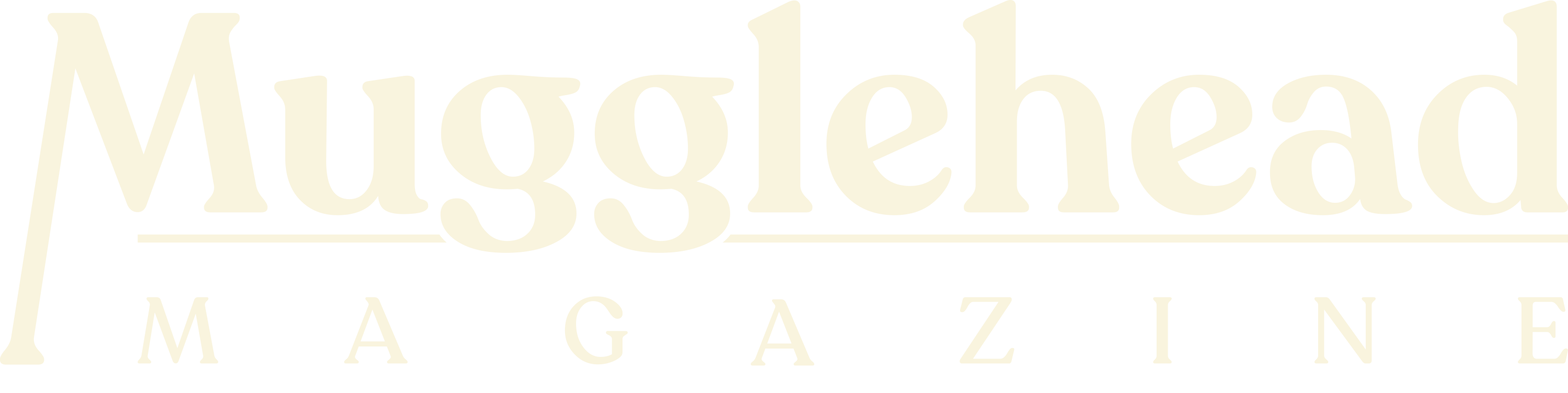