Using massive troves of data as the primary means of producing top-notch large language models (LLMs) is not necessarily the answer, according to new research from leading specialists.
Researchers from high-ranking universities like Carnegie Mellon, Stanford, Princeton and Harvard now think that overtraining AI models can be disastrous, ultimately making them more difficult to tweak and weakening their overall performance.
A group of them, led by Carnegie Mellon’s machine learning PhD student Jacob M. Springer, published a study titled ‘Overtrained Language Models Are Harder to Fine-Tune’ on Friday.
“In this work, we uncovered a surprising trend: contrary to common belief, longer pre-training does not always lead to better post-trained models,” the authors said. They refer to this phenomenon as “catastrophic overtraining” and say it is a frequent issue in today’s industry climate. The researchers claim to have definitively proved this in the second section of their extensive assessment (see link above).
“We demonstrate the prevalence of catastrophic overtraining across existing language models and tasks, showing that longer pre-training can degrade performance after instruction tuning and multimodal fine-tuning.”
For lead author Springer, fine-tuning AI models is currently a key focus as he completes his education. He has made several contributions to multiple artificial intelligence studies.
“Most recently, I have been thinking about how to train models that are easily and robustly fine-tuned to perform new tasks by design, and especially how optimization can influence this,” he explained. Springer says he is very excited about solving mysteries in machine learning. Him and his team have provided valuable insights to AI developers through their research.
Training with more data = better LLMs, right? 🚨
False! Scaling language models by adding more pre-training data can decrease your performance after post-training!
Introducing "catastrophic overtraining." 🥁🧵+arXiv 👇
1/9 pic.twitter.com/TpCDgZ862C
— Jacob Springer (@jacspringer) March 26, 2025
Read more: ChatGPT’s Japanese anime filter causes outrage; ‘melts’ OpenAI GPUs
Read more: Bowdoin College gets largest donation in history to launch AI research initiative
AI2 model validates ‘catastrophic overtraining’ implications
One of the key findings of their lengthy research paper was centred around the assessment of an LLM from the Allen Institute for AI (AI2).
They examined two different versions of the Seattle-based non-profit research school’s OLMo-1B model that had been trained with varying quantities of data. This LLM was released last fall.
“The instruction-tuned OLMo-1B model pre-trained on 3 trillion tokens [data] leads to over 2 per cent worse performance on multiple standard LLM benchmarks than its 2.3 trillion token counterpart,” the investigators specified.
Springer’s team says this is typical, not unusual.
GPT-4o from OpenAI, Anthropic’s Claude 3.7 Sonnet, Gemini 2.0 from Google, Elon Musk’s Grok 3 and DeepSeek R-1 are a handful of the world’s most advanced LLMs. Though imperfect and subject to “hallucinations” periodically, these programs serve as valuable research tools with multiple capabilities.
rowan@mugglehead.com
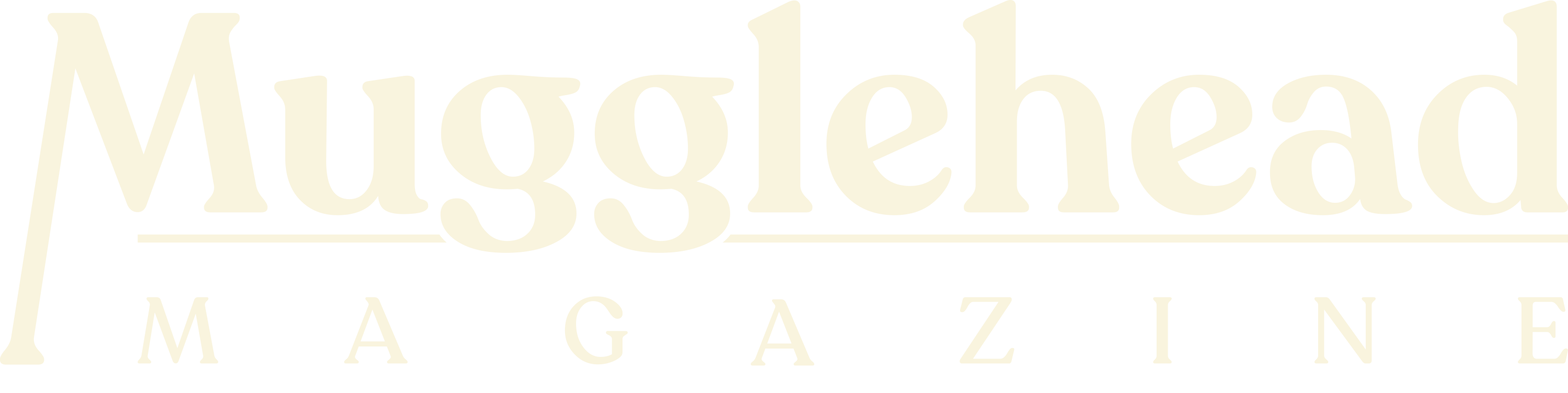